- Optimizing oil field development by clarifying the origin of dolomite reservoir rocks
- Application of machine learning in subsurface evaluation
- Application of distributed acoustic sensing (DAS) technology for subsurface monitoring
Optimizing oil field development by clarifying the origin of dolomite reservoir rocks
Dolomite is a commonly found mineral on earth. It plays a significant role in making up hydrocarbon reservoirs.
Dolomite has been the focus of academic study for a long time, but its origin is still largely unknown because experimental studies to produce dolomite at room temperature and pressure have not been successful.
Multiple models have been proposed for the formation process of dolomite. The accuracy in evaluating a carbonate reservoir property depends on the model that is selected. Predicting dolomite reservoir property is therefore a significant challenge in petroleum field development.
INPEX is focusing on the dolomite that forms reservoir rocks in a giant oil field offshore Abu Dhabi, United Arab Emirates. To determine the formation mechanism of these reservoir rocks, we are working together with a Japanese university combining their academic expertise in geochemical analysis with our specialty in reservoir rock characterization.
Through this study, we are targeting the efficient recovery of hydrocarbons by optimizing the location and design of wells based on the insight into the spatial distribution of the dolomite reservoir.
References
Yamamoto, K., Ottinger, G., Al Zinati, O., Takayanagi, H., Yamamoto, K. and Iryu, Y., 2018. Geochemical, petrographical, and petrophysical evaluations of a heterogeneous, stratiform dolomite from a Barremian oil field, offshore Abu Dhabi (United Arab Emirates).
Available at: https://archives.datapages.com/data/bulletns/2018/01jan/bltn17016/bltn17016.html
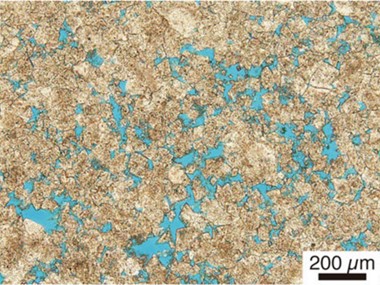
Application of machine learning in subsurface evaluation
Recent advances in technology have made it possible to obtain a substantial amount of relatively high-quality information on subsurface geology. However, the available information remains limited in quantity, and considerable manpower and cost are being spent on the improvement of subsurface reservoir models and mitigation of their uncertainties.
INPEX has been developing methodologies that employ machine learning, which has displayed remarkable advances in recent years. One example of such a technique is seismic inversion, which inversely analyzes the elastic property of subsurface rocks using seismic data. Another example is improvement of the quantitative seismic interpretation techniques, which analyze lithofacies and reservoir rock properties based on the results of seismic inversion.
In conventional seismic inversion, a model is manually built from existing wells to compensate low-frequency components missing in seismic data. This approach results in a wide range of flexibility in creating the models at locations away from wells and a high dependency of the inversion analysis on these models. Quantitative seismic interpretation is based on direct relationships among elastic properties, lithofacies and reservoir properties, which makes it challenging to incorporate known subsurface information like occurrence patterns of lithofacies into analysis. INPEX has been developing new analysis techniques that reduce the dependence on low-frequency models and allow the integration of known subsurface information by introducing machine learning.
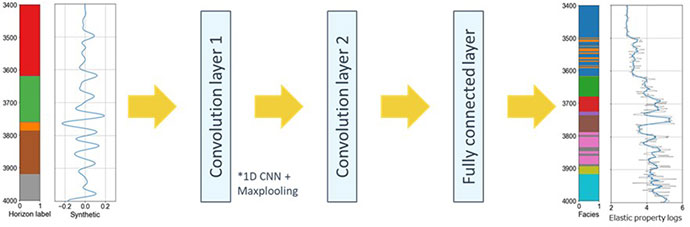
References
Desaki, S., Kobayashi, Y. and Fatwa A., n.d. Seismic inversion method using convolutional neural network: A case study from the Abadi field.
T. Yamatani and S. Desaki, (2023), "Lithofacies prediction from seismic data using deep learning: A case study from North West Shelf Australia," The Leading Edge 42: 773–781.
Application of distributed acoustic sensing (DAS) technology for subsurface monitoring
As carbon dioxide capture and storage (CCS) technology becomes more prevalent in the context of global warming countermeasures, understanding the state and behavior of carbon dioxide (CO2) stored underground is critically important. INPEX, in collaboration with the Japan Organization for Metals and Energy Security (JOGMEC), has conducted CO2EOR demonstration tests on the technology that enhances oil recovery through CO2 application on depleted onshore Oil and gas fields. Within this process and with an eye towards the future application to CCS projects, we have examined the practicality of distributed acoustic sensing (DAS) technology, the latest cutting-edge technology in subsurface monitoring.
DAS technology enables real-time, high-precision monitoring of the subsurface state by using optical fiber cables as sensors. In the CO2EOR demonstration tests, optical fiber cables for DAS were installed in two newly drilled wells, and seismic exploration data was collected simultaneously from both wells through seismic events induced on the surface. By integrating this data, we succeeded in capturing detailed subsurface structure images and constructing velocity models. Furthermore, in this demonstration,
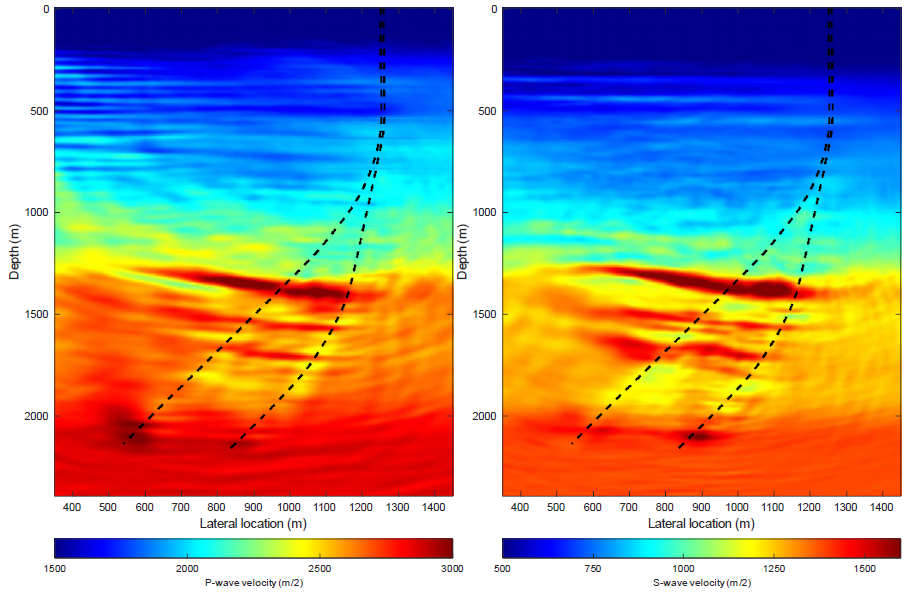
References
S. Nakayama, Y. Yamada, K. Fujita, T. Mouri, P. Bettinelli, J. Le Calvez, Y. Maehara, T. Mizuno, P. Armstrong, O. Podgornova, R. Bidyck, M. I. Rahmat, and D. Hill, (2024), "Diverse applications of DAS and their added values: A case study from Minami-aga pilot CCUS project, onshore Japan," The Leading Edge 43: 720–725.